Top Strategies to Optimize Your AI Search Experience for Maximum Efficiency
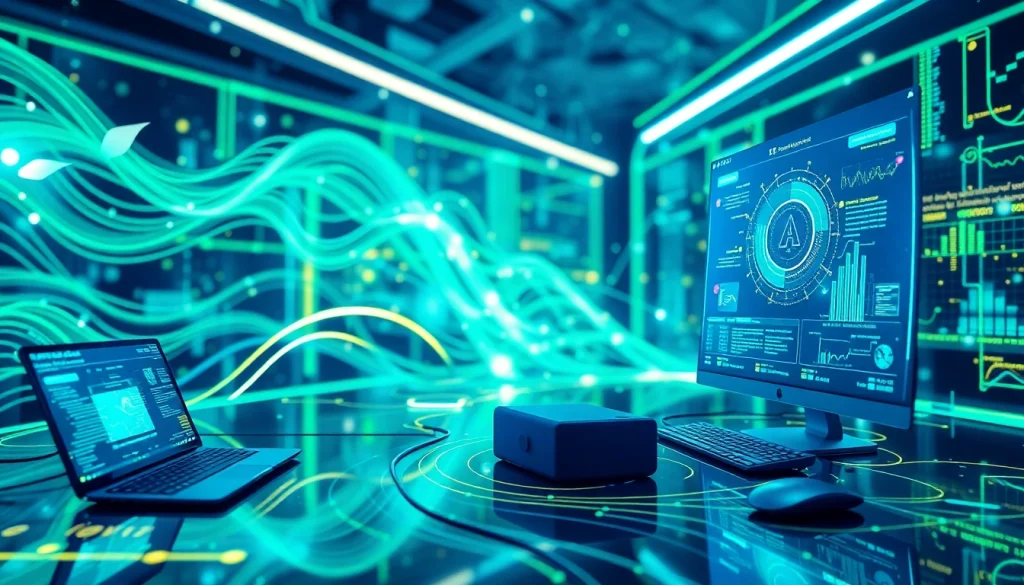
Understanding AI Search: A Breakdown
What is AI Search?
AI search refers to the application of artificial intelligence algorithms and techniques to enhance the search experience for users. This technology distinguishes itself from traditional keyword-based search engines by providing context-aware, efficient, and user-oriented results. AI search engines leverage natural language processing (NLP), machine learning, and advanced algorithms to analyze user queries and retrieve data from complex datasets. For instance, instead of merely matching keywords, an AI search engine strives to understand the intent behind a query, offering results that are more aligned with user expectations and needs.
The Evolution of Search Engines
The journey of search engines began in the early days of the internet with simple, keyword-based systems. As technology advanced, search engines like Google introduced PageRank algorithms, significantly improving search accuracy and relevance. However, the rapid expansion of information on the web created challenges in managing and retrieving data efficiently. The emergence of AI has revolutionized search engines, allowing for the incorporation of sophisticated models like GPT-3 and other neural networks. These models analyze data beyond mere keywords, focusing on context, user behavior, and machine learning to adapt over time.
Core Technologies Behind AI Search
The core technologies powering AI search solutions encompass several critical areas:
- Natural Language Processing (NLP): NLP enables machines to understand, interpret, and respond to human language in a valuable way. It’s crucial for deciphering user queries and providing relevant responses.
- Machine Learning: Through algorithms that learn from data, AI search engines adapt over time, continually improving their accuracy and relevance based on user interactions and feedback.
- Semantic Search: By understanding the intent behind user queries and the contextual relationship between words, semantic search differs significantly from traditional search methods, which rely strictly on keyword matching.
- Data Retrieval Techniques: Techniques such as vector space modeling and ranking algorithms ensure that the most pertinent data is returned to the user efficiently.
Key Benefits of AI Search Solutions
Improved Accuracy and Relevance
One of the primary advantages of AI search engines is their ability to deliver highly accurate and relevant results. Unlike traditional search engines, which often provide numerous results that may not pertain to the user’s specific needs, AI-driven systems focus on contextual understanding. By analyzing user history and preferences, these systems can predict which results will most likely meet the user’s intent, leading to fewer irrelevant results and more satisfied users.
Enhanced User Experience
Implementing AI in search operations enhances overall user experience significantly. AI search engines tend to offer a more conversational interface, enabling natural interactions similar to human conversations. Moreover, the personalization capabilities of AI lead to recommendations tailored to individual users, providing a unique experience that aligns with their preferences and previous interactions. This not only retains users but also promotes engagement.
Rapid Data Processing Capabilities
AI search engines excel in processing vast amounts of data quickly. Traditional systems can sometimes struggle with scalability, particularly during high-demand situations or with massive datasets. Conversely, AI-driven search solutions employ robust data processing methods such as parallel processing, which allows them to return results swiftly, even under heavy workloads—a significant advantage for businesses that depend on real-time search capabilities.
Challenges in Implementing AI Search
Data Privacy Issues
As organizations integrate AI search solutions, data privacy issues emerge as a critical concern. AI systems require extensive data to learn and adapt, including potentially sensitive user information. Organizations must navigate legal frameworks and ethical considerations, ensuring data is collected, stored, and processed in a manner that complies with regulations like GDPR. Balancing data utilization with privacy rights becomes essential in maintaining user trust.
Integration with Existing Systems
Another challenge lies in integrating AI search solutions with existing infrastructure. Organizations often have legacy systems that may not be compatible with new technologies. To avoid disruption, businesses must undertake thorough assessments and potentially overhaul their existing systems. This requires financial investment and considerable project management efforts to ensure a smooth transition.
Dependence on Quality Data
The efficiency of AI search engines hinges significantly on the quality of data fed into them. Poor, inaccurate, or biased data can lead to erroneous search results, damaging user trust in the system. Organizations need to invest in data cleansing and validation processes to ensure that their AI search tools function optimally. Additionally, they must implement feedback mechanisms to continuously improve the data quality over time.
Best Practices for Using AI Search Tools
Tailoring Search Algorithms
To maximize the effectiveness of AI search tools, organizations should focus on customizing search algorithms based on their unique datasets and user profiles. This involves analyzing search patterns and behaviors to tailor algorithms accordingly, resulting in more precise and relevant results. Utilizing A/B testing can help in evaluating algorithm effectiveness and making necessary adjustments for improved performance.
Continuous Learning and Adaptation
AI search systems should be built for continuous learning. By incorporating user feedback and behaviors, AI solutions can adapt to changing user needs. Establishing a closed-loop system where insights from user interactions are consistently fed back into the algorithms will ensure relevance over time, making the AI search tool an increasingly powerful asset for the organization.
User-Centric Design Principles
A user-centric approach is crucial for the design and development of AI search tools. This means involving users in the design process, gathering feedback, and iterating on designs based on real-world usage. By understanding the user experience from multiple viewpoints, developers can create interfaces that are not only functional but also appealing, leading to higher engagement levels.
Future Trends in AI Search Technology
Multimodal Search Capabilities
Looking ahead, one of the most exciting developments in AI search technology is the rise of multimodal search capabilities. This approach integrates various forms of input, including text, voice, and images. As users increasingly expect seamless interactions that blend different modalities, the search experience will become more intuitive. For example, users might query an AI search engine using voice commands while also providing a visual reference, leading to even richer search results.
Real-Time Data Access
The demand for real-time data access is growing, particularly in industries where timely information is critical. Future AI search systems are expected to integrate live updates from various sources, including social media, news outlets, and other platforms. This capability will enable organizations to provide users with the most current information, setting the stage for more informed decision-making and strategic operations.
AI Personalization Techniques
The future of AI search technology is likely to be heavily influenced by advanced personalization techniques. As AI search engines gather more data about user behaviors and preferences, they will become better at predicting what information users need before they even ask for it. This predictive capability will result in a more proactive search experience, with systems anticipating user needs and presenting relevant information seamlessly.